class: title-slide, left, bottom # Functional Programming with Purrr ---- ## **Birmingham R** ### **[Tom Jemmett][tj_email]** | Senior Healthcare Analyst ### January 2020 --- # About The Strategy Unit / Me .pull-left[ ***"Leading research, analysis and change from within the NHS"*** **The Strategy Unit** is a specialist **NHS** team, based in [**Midlands and Lancashire CSU**][mlcsu]. We focus on the application of high-quality, multi-disciplinary analytical work. Our team comes from diverse backgrounds. Our academic qualifications include maths, economics, history, natural sciences, medicine, sociology, business and management, psychology and political science. Our career and personal histories are just as varied. Our staff are **NHS** employees, animated by **NHS** values. **The Strategy Unit** covers all its costs through project funding. But this is driven by need, not what we can sell. Any surplus is recycled for public benefit. [<svg viewBox="0 0 576 512" style="height:1em;position:relative;display:inline-block;top:.1em;" xmlns="http://www.w3.org/2000/svg"> <path d="M528 0H48C21.5 0 0 21.5 0 48v320c0 26.5 21.5 48 48 48h192l-16 48h-72c-13.3 0-24 10.7-24 24s10.7 24 24 24h272c13.3 0 24-10.7 24-24s-10.7-24-24-24h-72l-16-48h192c26.5 0 48-21.5 48-48V48c0-26.5-21.5-48-48-48zm-16 352H64V64h448v288z"></path></svg>][su_web] | [<svg viewBox="0 0 512 512" style="height:1em;position:relative;display:inline-block;top:.1em;" xmlns="http://www.w3.org/2000/svg"> <path d="M464 64H48C21.49 64 0 85.49 0 112v288c0 26.51 21.49 48 48 48h416c26.51 0 48-21.49 48-48V112c0-26.51-21.49-48-48-48zm0 48v40.805c-22.422 18.259-58.168 46.651-134.587 106.49-16.841 13.247-50.201 45.072-73.413 44.701-23.208.375-56.579-31.459-73.413-44.701C106.18 199.465 70.425 171.067 48 152.805V112h416zM48 400V214.398c22.914 18.251 55.409 43.862 104.938 82.646 21.857 17.205 60.134 55.186 103.062 54.955 42.717.231 80.509-37.199 103.053-54.947 49.528-38.783 82.032-64.401 104.947-82.653V400H48z"></path></svg>][su_email] | [<svg viewBox="0 0 512 512" style="height:1em;position:relative;display:inline-block;top:.1em;" xmlns="http://www.w3.org/2000/svg"> <path d="M459.37 151.716c.325 4.548.325 9.097.325 13.645 0 138.72-105.583 298.558-298.558 298.558-59.452 0-114.68-17.219-161.137-47.106 8.447.974 16.568 1.299 25.34 1.299 49.055 0 94.213-16.568 130.274-44.832-46.132-.975-84.792-31.188-98.112-72.772 6.498.974 12.995 1.624 19.818 1.624 9.421 0 18.843-1.3 27.614-3.573-48.081-9.747-84.143-51.98-84.143-102.985v-1.299c13.969 7.797 30.214 12.67 47.431 13.319-28.264-18.843-46.781-51.005-46.781-87.391 0-19.492 5.197-37.36 14.294-52.954 51.655 63.675 129.3 105.258 216.365 109.807-1.624-7.797-2.599-15.918-2.599-24.04 0-57.828 46.782-104.934 104.934-104.934 30.213 0 57.502 12.67 76.67 33.137 23.715-4.548 46.456-13.32 66.599-25.34-7.798 24.366-24.366 44.833-46.132 57.827 21.117-2.273 41.584-8.122 60.426-16.243-14.292 20.791-32.161 39.308-52.628 54.253z"></path></svg>][su_twitter] | [<svg viewBox="0 0 496 512" style="height:1em;position:relative;display:inline-block;top:.1em;" xmlns="http://www.w3.org/2000/svg"> <path d="M165.9 397.4c0 2-2.3 3.6-5.2 3.6-3.3.3-5.6-1.3-5.6-3.6 0-2 2.3-3.6 5.2-3.6 3-.3 5.6 1.3 5.6 3.6zm-31.1-4.5c-.7 2 1.3 4.3 4.3 4.9 2.6 1 5.6 0 6.2-2s-1.3-4.3-4.3-5.2c-2.6-.7-5.5.3-6.2 2.3zm44.2-1.7c-2.9.7-4.9 2.6-4.6 4.9.3 2 2.9 3.3 5.9 2.6 2.9-.7 4.9-2.6 4.6-4.6-.3-1.9-3-3.2-5.9-2.9zM244.8 8C106.1 8 0 113.3 0 252c0 110.9 69.8 205.8 169.5 239.2 12.8 2.3 17.3-5.6 17.3-12.1 0-6.2-.3-40.4-.3-61.4 0 0-70 15-84.7-29.8 0 0-11.4-29.1-27.8-36.6 0 0-22.9-15.7 1.6-15.4 0 0 24.9 2 38.6 25.8 21.9 38.6 58.6 27.5 72.9 20.9 2.3-16 8.8-27.1 16-33.7-55.9-6.2-112.3-14.3-112.3-110.5 0-27.5 7.6-41.3 23.6-58.9-2.6-6.5-11.1-33.3 2.6-67.9 20.9-6.5 69 27 69 27 20-5.6 41.5-8.5 62.8-8.5s42.8 2.9 62.8 8.5c0 0 48.1-33.6 69-27 13.7 34.7 5.2 61.4 2.6 67.9 16 17.7 25.8 31.5 25.8 58.9 0 96.5-58.9 104.2-114.8 110.5 9.2 7.9 17 22.9 17 46.4 0 33.7-.3 75.4-.3 83.6 0 6.5 4.6 14.4 17.3 12.1C428.2 457.8 496 362.9 496 252 496 113.3 383.5 8 244.8 8zM97.2 352.9c-1.3 1-1 3.3.7 5.2 1.6 1.6 3.9 2.3 5.2 1 1.3-1 1-3.3-.7-5.2-1.6-1.6-3.9-2.3-5.2-1zm-10.8-8.1c-.7 1.3.3 2.9 2.3 3.9 1.6 1 3.6.7 4.3-.7.7-1.3-.3-2.9-2.3-3.9-2-.6-3.6-.3-4.3.7zm32.4 35.6c-1.6 1.3-1 4.3 1.3 6.2 2.3 2.3 5.2 2.6 6.5 1 1.3-1.3.7-4.3-1.3-6.2-2.2-2.3-5.2-2.6-6.5-1zm-11.4-14.7c-1.6 1-1.6 3.6 0 5.9 1.6 2.3 4.3 3.3 5.6 2.3 1.6-1.3 1.6-3.9 0-6.2-1.4-2.3-4-3.3-5.6-2z"></path></svg>][su_github] | [<svg viewBox="0 0 448 512" style="height:1em;position:relative;display:inline-block;top:.1em;" xmlns="http://www.w3.org/2000/svg"> <path d="M416 32H31.9C14.3 32 0 46.5 0 64.3v383.4C0 465.5 14.3 480 31.9 480H416c17.6 0 32-14.5 32-32.3V64.3c0-17.8-14.4-32.3-32-32.3zM135.4 416H69V202.2h66.5V416zm-33.2-243c-21.3 0-38.5-17.3-38.5-38.5S80.9 96 102.2 96c21.2 0 38.5 17.3 38.5 38.5 0 21.3-17.2 38.5-38.5 38.5zm282.1 243h-66.4V312c0-24.8-.5-56.7-34.5-56.7-34.6 0-39.9 27-39.9 54.9V416h-66.4V202.2h63.7v29.2h.9c8.9-16.8 30.6-34.5 62.9-34.5 67.2 0 79.7 44.3 79.7 101.9V416z"></path></svg>][su_linkedin] ] .pull-right[ **Tom Jemmett** *Senior Healthcare Analyst* [thomas.jemmett@nhs.net][tj_email] - 10+ years experience within the NHS as a data analyst - BSc Computer Science and Pure Mathematics ([Open University][open_uni]) - [MBCS][bcs]/[AMIMA][ima] - Active member of NHS-R community - Senior Fellow of NHS-R academy - [AphA][apha] member, West Midlands branch champion [<svg viewBox="0 0 512 512" style="height:1em;position:relative;display:inline-block;top:.1em;" xmlns="http://www.w3.org/2000/svg"> <path d="M464 64H48C21.49 64 0 85.49 0 112v288c0 26.51 21.49 48 48 48h416c26.51 0 48-21.49 48-48V112c0-26.51-21.49-48-48-48zm0 48v40.805c-22.422 18.259-58.168 46.651-134.587 106.49-16.841 13.247-50.201 45.072-73.413 44.701-23.208.375-56.579-31.459-73.413-44.701C106.18 199.465 70.425 171.067 48 152.805V112h416zM48 400V214.398c22.914 18.251 55.409 43.862 104.938 82.646 21.857 17.205 60.134 55.186 103.062 54.955 42.717.231 80.509-37.199 103.053-54.947 49.528-38.783 82.032-64.401 104.947-82.653V400H48z"></path></svg>][tj_email] | [<svg viewBox="0 0 512 512" style="height:1em;position:relative;display:inline-block;top:.1em;" xmlns="http://www.w3.org/2000/svg"> <path d="M459.37 151.716c.325 4.548.325 9.097.325 13.645 0 138.72-105.583 298.558-298.558 298.558-59.452 0-114.68-17.219-161.137-47.106 8.447.974 16.568 1.299 25.34 1.299 49.055 0 94.213-16.568 130.274-44.832-46.132-.975-84.792-31.188-98.112-72.772 6.498.974 12.995 1.624 19.818 1.624 9.421 0 18.843-1.3 27.614-3.573-48.081-9.747-84.143-51.98-84.143-102.985v-1.299c13.969 7.797 30.214 12.67 47.431 13.319-28.264-18.843-46.781-51.005-46.781-87.391 0-19.492 5.197-37.36 14.294-52.954 51.655 63.675 129.3 105.258 216.365 109.807-1.624-7.797-2.599-15.918-2.599-24.04 0-57.828 46.782-104.934 104.934-104.934 30.213 0 57.502 12.67 76.67 33.137 23.715-4.548 46.456-13.32 66.599-25.34-7.798 24.366-24.366 44.833-46.132 57.827 21.117-2.273 41.584-8.122 60.426-16.243-14.292 20.791-32.161 39.308-52.628 54.253z"></path></svg>][tj_twitter] | [<svg viewBox="0 0 496 512" style="height:1em;position:relative;display:inline-block;top:.1em;" xmlns="http://www.w3.org/2000/svg"> <path d="M165.9 397.4c0 2-2.3 3.6-5.2 3.6-3.3.3-5.6-1.3-5.6-3.6 0-2 2.3-3.6 5.2-3.6 3-.3 5.6 1.3 5.6 3.6zm-31.1-4.5c-.7 2 1.3 4.3 4.3 4.9 2.6 1 5.6 0 6.2-2s-1.3-4.3-4.3-5.2c-2.6-.7-5.5.3-6.2 2.3zm44.2-1.7c-2.9.7-4.9 2.6-4.6 4.9.3 2 2.9 3.3 5.9 2.6 2.9-.7 4.9-2.6 4.6-4.6-.3-1.9-3-3.2-5.9-2.9zM244.8 8C106.1 8 0 113.3 0 252c0 110.9 69.8 205.8 169.5 239.2 12.8 2.3 17.3-5.6 17.3-12.1 0-6.2-.3-40.4-.3-61.4 0 0-70 15-84.7-29.8 0 0-11.4-29.1-27.8-36.6 0 0-22.9-15.7 1.6-15.4 0 0 24.9 2 38.6 25.8 21.9 38.6 58.6 27.5 72.9 20.9 2.3-16 8.8-27.1 16-33.7-55.9-6.2-112.3-14.3-112.3-110.5 0-27.5 7.6-41.3 23.6-58.9-2.6-6.5-11.1-33.3 2.6-67.9 20.9-6.5 69 27 69 27 20-5.6 41.5-8.5 62.8-8.5s42.8 2.9 62.8 8.5c0 0 48.1-33.6 69-27 13.7 34.7 5.2 61.4 2.6 67.9 16 17.7 25.8 31.5 25.8 58.9 0 96.5-58.9 104.2-114.8 110.5 9.2 7.9 17 22.9 17 46.4 0 33.7-.3 75.4-.3 83.6 0 6.5 4.6 14.4 17.3 12.1C428.2 457.8 496 362.9 496 252 496 113.3 383.5 8 244.8 8zM97.2 352.9c-1.3 1-1 3.3.7 5.2 1.6 1.6 3.9 2.3 5.2 1 1.3-1 1-3.3-.7-5.2-1.6-1.6-3.9-2.3-5.2-1zm-10.8-8.1c-.7 1.3.3 2.9 2.3 3.9 1.6 1 3.6.7 4.3-.7.7-1.3-.3-2.9-2.3-3.9-2-.6-3.6-.3-4.3.7zm32.4 35.6c-1.6 1.3-1 4.3 1.3 6.2 2.3 2.3 5.2 2.6 6.5 1 1.3-1.3.7-4.3-1.3-6.2-2.2-2.3-5.2-2.6-6.5-1zm-11.4-14.7c-1.6 1-1.6 3.6 0 5.9 1.6 2.3 4.3 3.3 5.6 2.3 1.6-1.3 1.6-3.9 0-6.2-1.4-2.3-4-3.3-5.6-2z"></path></svg>][tj_github] | [<svg viewBox="0 0 448 512" style="height:1em;position:relative;display:inline-block;top:.1em;" xmlns="http://www.w3.org/2000/svg"> <path d="M416 32H31.9C14.3 32 0 46.5 0 64.3v383.4C0 465.5 14.3 480 31.9 480H416c17.6 0 32-14.5 32-32.3V64.3c0-17.8-14.4-32.3-32-32.3zM135.4 416H69V202.2h66.5V416zm-33.2-243c-21.3 0-38.5-17.3-38.5-38.5S80.9 96 102.2 96c21.2 0 38.5 17.3 38.5 38.5 0 21.3-17.2 38.5-38.5 38.5zm282.1 243h-66.4V312c0-24.8-.5-56.7-34.5-56.7-34.6 0-39.9 27-39.9 54.9V416h-66.4V202.2h63.7v29.2h.9c8.9-16.8 30.6-34.5 62.9-34.5 67.2 0 79.7 44.3 79.7 101.9V416z"></path></svg>][tj_linkedin] ] --- class: inverse ## Purrr Purrr is a package that aims to provide "A complete and consistent functional programming toolkit for R." Functional Programming is a [Declarative Programming Paradigm][1] that treats computation as the evaluation of [Mathematical Functions][2]. From a [Mango blog post][6] > FP is the process of writing code in a structured way and through functions > remove code duplications and redundancies. In effect, computations or > evaluations are treated as mathematical functions and the output of a function > only depends on the values of its inputs – known as arguments. FP ensures that > any side-effects such as changes in state do not affect the expected output > such that if you call the same function twice with the same arguments the > function returns the same output. As of version 0.3.3 there are some 177 functions exported by purrr! Fortunately, most of these functions are variants of one another, and some 65 of these functions all fall under the same family of "map" functions. --- ## map .pull-left[ map is a function that takes a vector (or list) and another function for which each item from the input vector (or list) is evaluated against. ```r map <- function(.x, .f, ...) ``` map returns a list - but you can use the map_ variants if you want to return a specific type of data as a vector: * map_chr for a character vector * map_dbl for a numeric vector * map_lgl for a logical (`TRUE`/`FALSE`) vector * map_int for an integer vector * map_raw for a raw rector * map_dfr if your function returns dataframes and you want to bind by rows (rbind) * map_dfc if your function returns dataframes and you want to bind by columns (cbind) ] .pull-right[ 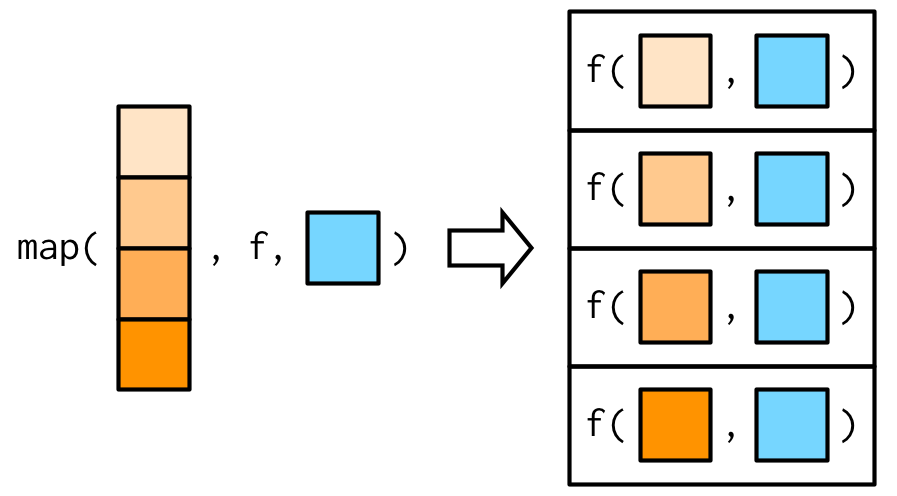 source: [Wickham, H. (2020). 9 Functionals | Advanced R.][3] map is very similar to the apply family of functions in Base R, but provide a much simpler and more consistent programming interface. ] --- ## simple map examples .pull-left[ Let's say we have a function ```r fn <- function(x, a) { x^2 + a } ``` We can use map to iterate over the numbers from 1 to 5 and return the results as a list. ```r map(1:5, fn, 3) ``` ``` ## [[1]] ## [1] 4 ## ## [[2]] ## [1] 7 ## ## [[3]] ## [1] 12 ## ## [[4]] ## [1] 19 ## ## [[5]] ## [1] 28 ``` ] .pull-right[ If we want to simplify the results, we can use the map_dbl function, which will give us the results as a numeric vector. We can also define functions inside of map. We can either write out the full function syntax, or we can use a formula syntax like: ```r map_dbl(1:5, ~.x^2 + 3) ``` ``` ## [1] 4 7 12 19 28 ``` in the formula syntax the argument is called `.x`. .small[ of course, if your function is vectorised you could just call `fn(1:5, 3)`... ] ] --- ## reading in csv's from a folder ```r files <- dir(here::here("data", "ae_attendances"), "^\\d{4}-\\d{2}-\\d{2}.csv$", full.names = TRUE) # set the name of each item in the vector to be the date part of the file names(files) <- files %>% str_extract("\\d{4}-\\d{2}-\\d{2}.csv") # map over the list of files, read each csv, add a column called "file" with the # value of the name of the item. ae_attendances <- map_dfr(files, read_csv, col_types = "Dccddd", .id = "file") knitr::kable(head(ae_attendances, 5), format="html") ``` <table> <thead> <tr> <th style="text-align:left;"> file </th> <th style="text-align:left;"> period </th> <th style="text-align:left;"> org_code </th> <th style="text-align:left;"> type </th> <th style="text-align:right;"> attendances </th> <th style="text-align:right;"> breaches </th> <th style="text-align:right;"> admissions </th> </tr> </thead> <tbody> <tr> <td style="text-align:left;"> 2016-04-01.csv </td> <td style="text-align:left;"> 2016-04-01 </td> <td style="text-align:left;"> RF4 </td> <td style="text-align:left;"> 1 </td> <td style="text-align:right;"> 18788 </td> <td style="text-align:right;"> 4082 </td> <td style="text-align:right;"> 4074 </td> </tr> <tr> <td style="text-align:left;"> 2016-04-01.csv </td> <td style="text-align:left;"> 2016-04-01 </td> <td style="text-align:left;"> RF4 </td> <td style="text-align:left;"> 2 </td> <td style="text-align:right;"> 561 </td> <td style="text-align:right;"> 5 </td> <td style="text-align:right;"> 0 </td> </tr> <tr> <td style="text-align:left;"> 2016-04-01.csv </td> <td style="text-align:left;"> 2016-04-01 </td> <td style="text-align:left;"> RF4 </td> <td style="text-align:left;"> other </td> <td style="text-align:right;"> 2685 </td> <td style="text-align:right;"> 17 </td> <td style="text-align:right;"> 0 </td> </tr> <tr> <td style="text-align:left;"> 2016-04-01.csv </td> <td style="text-align:left;"> 2016-04-01 </td> <td style="text-align:left;"> R1H </td> <td style="text-align:left;"> 1 </td> <td style="text-align:right;"> 27396 </td> <td style="text-align:right;"> 5099 </td> <td style="text-align:right;"> 6424 </td> </tr> <tr> <td style="text-align:left;"> 2016-04-01.csv </td> <td style="text-align:left;"> 2016-04-01 </td> <td style="text-align:left;"> R1H </td> <td style="text-align:left;"> 2 </td> <td style="text-align:right;"> 700 </td> <td style="text-align:right;"> 5 </td> <td style="text-align:right;"> 0 </td> </tr> </tbody> </table> --- ## map2: map over two lists .pull-left[ Let's generate a table with random binomially distributed values: ```r df <- tibble(i = 1:20) %>% mutate(N = rpois(max(i), 100)) %>% mutate(p = map_dbl(N, rbinom, n = 1, prob = 0.75)) knitr::kable(head(df, 5), format="html") ``` <table> <thead> <tr> <th style="text-align:right;"> i </th> <th style="text-align:right;"> N </th> <th style="text-align:right;"> p </th> </tr> </thead> <tbody> <tr> <td style="text-align:right;"> 1 </td> <td style="text-align:right;"> 102 </td> <td style="text-align:right;"> 79 </td> </tr> <tr> <td style="text-align:right;"> 2 </td> <td style="text-align:right;"> 89 </td> <td style="text-align:right;"> 67 </td> </tr> <tr> <td style="text-align:right;"> 3 </td> <td style="text-align:right;"> 97 </td> <td style="text-align:right;"> 75 </td> </tr> <tr> <td style="text-align:right;"> 4 </td> <td style="text-align:right;"> 113 </td> <td style="text-align:right;"> 88 </td> </tr> <tr> <td style="text-align:right;"> 5 </td> <td style="text-align:right;"> 103 </td> <td style="text-align:right;"> 84 </td> </tr> </tbody> </table> ] .pull-right[ we can use `map2` to iterate over the pairs of numbers in the `N` and `p` columns, and use the `BinomCI` function (from `DescTools`) to calculate confidence intervals. ```r df %>% mutate(confint = map2(p, N, BinomCI) %>% map(as_tibble)) %>% unnest(cols = "confint") %>% head(5) %>% knitr::kable(format = "html") ``` <table> <thead> <tr> <th style="text-align:right;"> i </th> <th style="text-align:right;"> N </th> <th style="text-align:right;"> p </th> <th style="text-align:right;"> est </th> <th style="text-align:right;"> lwr.ci </th> <th style="text-align:right;"> upr.ci </th> </tr> </thead> <tbody> <tr> <td style="text-align:right;"> 1 </td> <td style="text-align:right;"> 102 </td> <td style="text-align:right;"> 79 </td> <td style="text-align:right;"> 0.7745098 </td> <td style="text-align:right;"> 0.6843102 </td> <td style="text-align:right;"> 0.8447831 </td> </tr> <tr> <td style="text-align:right;"> 2 </td> <td style="text-align:right;"> 89 </td> <td style="text-align:right;"> 67 </td> <td style="text-align:right;"> 0.7528090 </td> <td style="text-align:right;"> 0.6539796 </td> <td style="text-align:right;"> 0.8307176 </td> </tr> <tr> <td style="text-align:right;"> 3 </td> <td style="text-align:right;"> 97 </td> <td style="text-align:right;"> 75 </td> <td style="text-align:right;"> 0.7731959 </td> <td style="text-align:right;"> 0.6803956 </td> <td style="text-align:right;"> 0.8451819 </td> </tr> <tr> <td style="text-align:right;"> 4 </td> <td style="text-align:right;"> 113 </td> <td style="text-align:right;"> 88 </td> <td style="text-align:right;"> 0.7787611 </td> <td style="text-align:right;"> 0.6937769 </td> <td style="text-align:right;"> 0.8454152 </td> </tr> <tr> <td style="text-align:right;"> 5 </td> <td style="text-align:right;"> 103 </td> <td style="text-align:right;"> 84 </td> <td style="text-align:right;"> 0.8155340 </td> <td style="text-align:right;"> 0.7297734 </td> <td style="text-align:right;"> 0.8786046 </td> </tr> </tbody> </table> ] --- ## Other map functions .pull-left[ #### pmap If you have more than 2 items you need to map over, then you want pmap: Your input must be a list, and each item in the list must contain the same number of items. ```r list(x = 1:3, y = 4:6, z = 7:9) %>% pmap_dbl(function(x, y, z) x^y+z) ``` ``` ## [1] 8 40 738 ``` If you pass in a dataframe then it will run a function for each row. The functions arguments need to match the name of the items in the list/columns in the dataframe rather than by position. ] .pull-right[ #### imap imap works just like map, except it adds an "index" value, so your function call will be function(.x, .i). #### walk walk/walk2/pwalk/iwalk works calls the function for it's side effects, but it returns it's original input. Useful for things like calling `rmarkdown::render`. #### modify For when your output is going to be the same type as your input. Can be awkward as some functions subtly change the data type (e.g. integers cast to numerics). ] --- ## Other useful functions from purrr .pull-left[ #### Composing multiple functions together You can chain multiple map's together, for example: ```r 1:5 %>% map_dbl(~.x^2) %>% map_dbl(~.x+3) ``` ``` ## [1] 4 7 12 19 28 ``` the compose function allows us to combine functions together. ```r map_dbl(1:5, compose(~.x^2, ~.x+3, .dir="forward")) ``` ``` ## [1] 4 7 12 19 28 ``` by default, compose works backwards, like the mathematical "dot" composition, you can change this by setting `.dir="forward"`. ] .pull-right[ #### Partial application We can partially apply functions arguments to create a new, simpler function. ```r mean_na <- partial(mean, na.rm = TRUE) mean_na(c(1:5, NA)) ``` ``` ## [1] 3 ``` a good example of when partial can be useful is when used in combination with compose: if we want to pass additional arguments to the second or 3 function in the chain, then we will need to set these arguments with `partial` during the `compose` call ```r compose(function_1, partial(function_2, arg = value), function_3) ``` ] --- ## Using map to calculate confidence intervals in a tibble .pull-left[ Below we take the `ae_attendances` data and sum to get just the monthly performance values. We use the `BinomCI` function from the `DescTools` package to calculate the percentage of attendances that "breached" the 4 hour target, along with the 95% confidence intervals. We wouldn't normally be able to call this function inside a tidy pipe because it's expecting a single value for the `x` and `n` arguments. Using map2 we run the function once for each pair of values. We compose with as_tibble to convert the results into a table - this helps us in the next step to unnest this column. ] .pull-right[ ```r ae_attendances %>% group_by(period) %>% summarise_at(vars(attendances, breaches), sum) %>% mutate(ci = map2(breaches, attendances, compose(as_tibble, BinomCI))) %>% unnest(cols = c(ci)) %>% select_if(negate(is.list)) %>% mutate_at(vars(est:upr.ci), ~1-.x) %>% select(period, est, lwr.ci, upr.ci) %>% head(3) %>% knitr::kable(format = "html") ``` <table> <thead> <tr> <th style="text-align:left;"> period </th> <th style="text-align:right;"> est </th> <th style="text-align:right;"> lwr.ci </th> <th style="text-align:right;"> upr.ci </th> </tr> </thead> <tbody> <tr> <td style="text-align:left;"> 2016-04-01 </td> <td style="text-align:right;"> 0.9003513 </td> <td style="text-align:right;"> 0.9007800 </td> <td style="text-align:right;"> 0.8999209 </td> </tr> <tr> <td style="text-align:left;"> 2016-05-01 </td> <td style="text-align:right;"> 0.9027556 </td> <td style="text-align:right;"> 0.9031584 </td> <td style="text-align:right;"> 0.9023512 </td> </tr> <tr> <td style="text-align:left;"> 2016-06-01 </td> <td style="text-align:right;"> 0.9055994 </td> <td style="text-align:right;"> 0.9060081 </td> <td style="text-align:right;"> 0.9051892 </td> </tr> </tbody> </table> ] --- class: inverse ## Further Reading * [Advanced R][3] contains an excellent section on Functional Programming * [R4DS][4] functions section is well worth reading also if you haven't created many of your own functions * [Herding Cats with List Columns and Purrr][5] shows some more advanced use cases for using purrr * [To Purrr or not to purrr][6] goes into a bit more detail about why you should care about functional programming * [furrr][7] is a package that provides future-compatible versions of the map functions that allow you to quickly parallelise your code <!-- urls etc. --> [tj_email]: mailto:thomas.jemmett@nhs.net [tj_twitter]: https://twitter.com/tomjemmett [tj_github]: https://github.com/tomjemmett [tj_linkedin]: https://www.linkedin.com/in/tom-jemmett-3872a8159/ [su_web]: https://strategyunitwm.nhs.uk/ [su_email]: mailto:strategy.unit@nhs.net [su_twitter]: https://twitter.com/strategy_unit [su_github]: https://github.com/The-Strategy-Unit/ [su_linkedin]: https://www.linkedin.com/company/the-strategy-unit/ [mlcsu]: https://www.midlandsandlancashirecsu.nhs.uk/ [open_uni]: https://www.open.ac.uk/ [bcs]: https://www.bcs.org/ [ima]: https://ima.org.uk/ [apha]: https://www.aphanalysts.org/ [1]:https://en.wikipedia.org/wiki/Declarative_programming [2]:https://en.wikipedia.org/wiki/Function_(mathematics) [3]:https://adv-r.hadley.nz/functionals.html [4]:https://r4ds.had.co.nz/functions.html [5]:http://www.josephtpowers.com/post/herding-cats-with-list-columns-and-purrr/ [6]:https://www.mango-solutions.com/to-purrr-or-not-to-purrr/ [7]:https://github.com/DavisVaughan/furrr